AI
Security
Reviews
Helping you responsibly build solutions by identifying security gaps in your AI implementation.
Our Experience
Reviewing and Testing AI Models
TESTING
We have tested applications integrated with proprietary AI models before the mainstream adoption of AI.
Our experience with penetration testing AI models has spanned proprietary models, adapted open-source models, such as those from repositories like HuggingFace, and models built using popular technologies such as ChatGPT.
Further, our methodology for penetration testing against AI models is influenced by our first-hand experience of delivering red team engagements against clients who have deployed an AI model for a commercial purpose.
ARCHITECTURE REVIEWS
We have reviewed the overall architecture of the system which integrates with the AI model. This includes third-party AI integrations as well as those developed and managed internally. Our reviews have focused on ensuring layered defences exist across the system architecture such that the appropriate level of security is afforded to the AI model.
SOURCE-CODE REVIEWS
In tangent with penetration tests against AI models, where source-code has been available, we have performed a line-by-line audit of a large volume of models. These reviews are performed in a hybrid fashion with some automated analysis, but the onus being placed on manual reviews by Wilbourne's in-house secure code development consultants.
DEVSECOPS REVIEWS
Wilbourne is often asked by our clients to help modernise their DevSecOps capability. A DevSecOps improvement review naturally lends itself to securing the development and integration of an AI model. We have experience delivering DevSecOps reviews for solutions wholly built on AI as well as existing solutions which have recently been coupled with an AI component.
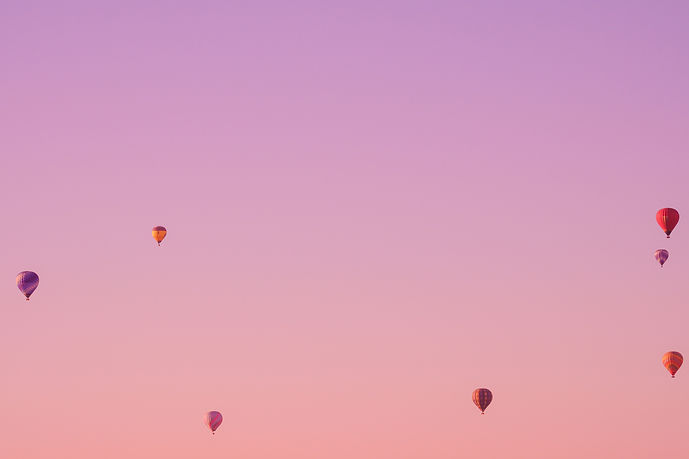
How does Wilbourne ensure appropriate coverage during an AI review?
AI models have achieved unprecedented growth and are continuing to grow at an exponential rate, which renders a standardised testing strategy to be inappropriate for some models. As a result, where Wilbourne comes across frontier or proprietary models, we work closely with AI developers and engineers to develop a bespoke testing methodology to cover such additional functionality.
This malleable approach reduces the risk of functionality not being tested and vulnerabilities not being discovered in models. Wilbourne's approach ensures appropriate coverage of the model’s capabilities.
What is the process of performing an AI Security Review with Wilbourne?
Given the nuances of the world of AI, we will collaborate with you to understand the Total Product Lifecycle (TPL) of the system in which your model sits within. This will involve covering the following three areas, before shaping the engagement to match your needs and starting technical delivery.​​​​​
If you already have a clear view of the support you would like from Wilbourne, this process can be streamlined further, and you can quickly leverage our technical and strategic expertise to begin delivery of the engagement you envision.